Lifeng Liu resumes & CV records
Resumes
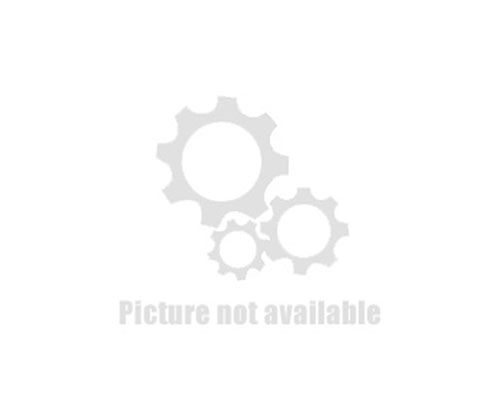
Architect
Location:
Arlington, MA
Industry:
Computer Software
Work:
Futurewei Technologies
Architect Cognex Corporation Oct 1, 2005 - Dec 2016
Software Engineer Center For Neurological Imaging Brigham and Women’s Hospital May 2003 - Oct 2005
Computer Scientist Mdol 2000 - 2002
Senior Software Engineer
Architect Cognex Corporation Oct 1, 2005 - Dec 2016
Software Engineer Center For Neurological Imaging Brigham and Women’s Hospital May 2003 - Oct 2005
Computer Scientist Mdol 2000 - 2002
Senior Software Engineer
Education:
Boston University 1986 - 2001
Boston University 1996 - 2001
Tsinghua University 1987 - 1996
Masters, Bachelors, Bachelor of Engineering, Engineering
Boston University 1996 - 2001
Tsinghua University 1987 - 1996
Masters, Bachelors, Bachelor of Engineering, Engineering
Skills:
Algorithms, Image Processing, Software Engineering, Software Development, Computer Vision, Machine Learning, Pattern Recognition, C++, Java
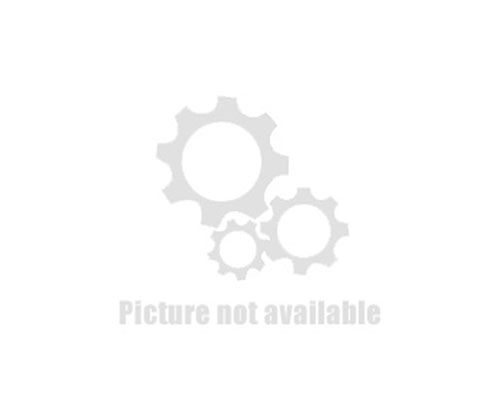
Lifeng Liu
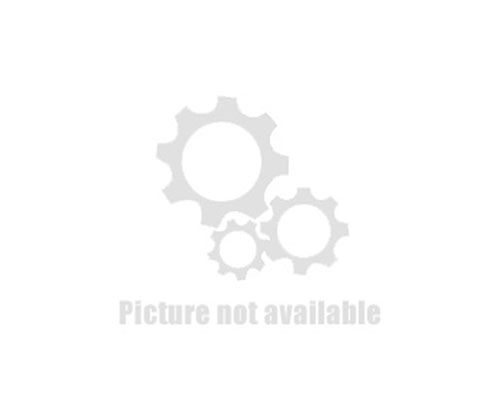
Lifeng Liu
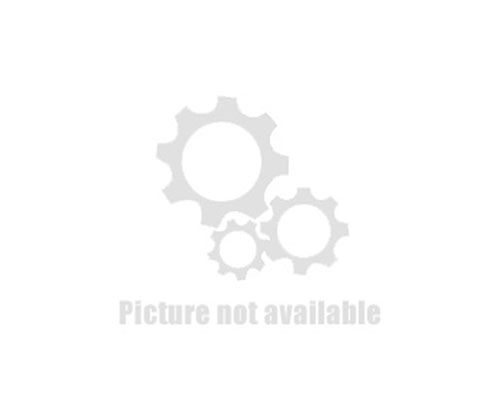
Lifeng Liu
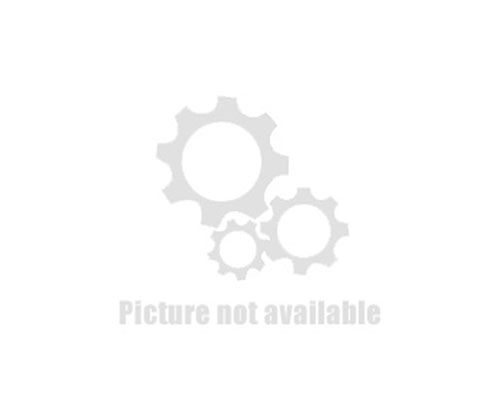