Xian Xu resumes & CV records
Resumes
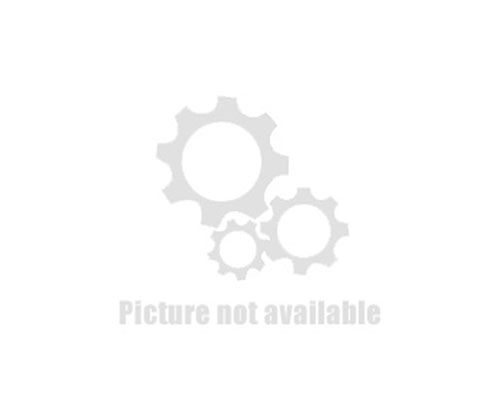
Cupertino, CA
San Jose, CA
Sunnyvale, CA
Sandusky, IA
Austin, TX
Camarillo, CA
10518 Mcclellan Pl, Cupertino, CA 95014
Mentions for Xian Zhu Xu
NOTICE: You may not use BackgroundCheck or the information it provides to make decisions about employment, credit, housing or any other purpose that would require Fair Credit Reporting Act (FCRA) compliance. BackgroundCheck is not a Consumer Reporting Agency (CRA) as defined by the FCRA and does not provide consumer reports.