Xu Miao resumes & CV records
Resumes
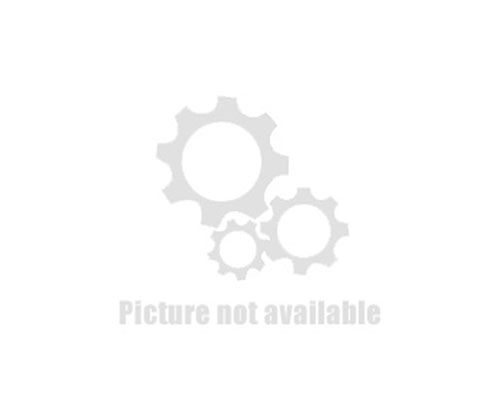
Xu Miao
Industry:
Computer Software
Education:
Nanjing University 2004 - 2009
Los Altos, CA
Sunnyvale, CA
6017 25Th Ave NE, Seattle, WA 98115 206-5253901 206-9859418
Berkeley, CA
Mentions for Xu U Miao
NOTICE: You may not use BackgroundCheck or the information it provides to make decisions about employment, credit, housing or any other purpose that would require Fair Credit Reporting Act (FCRA) compliance. BackgroundCheck is not a Consumer Reporting Agency (CRA) as defined by the FCRA and does not provide consumer reports.